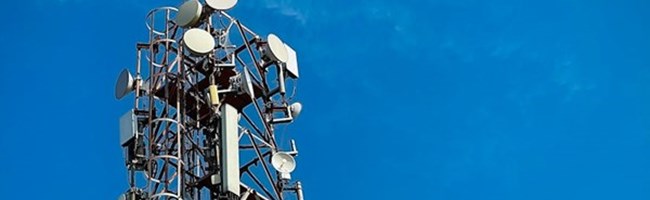
Data quality is key
Poor data quality can lead to poor decisions, thus it is crucial to know and understand the quality of your data.
As part of the project, we studied the data quality of sensor networks. So far, we studied methods to assess the quality of different aspects in datasets, in particular sensor network datasets. Via a literature study, many different aspects of data quality have been explored. Some aspects are generally applicable, such as data accuracy, completeness i.e. how many datapoints are missing, and timeliness i.e. how recent is the data obtained.
Redundancy
Other aspects are particularly relevant for sensor networks, such as redundancy, i.e. how robust is the network when one or more sensors are malfunctioning. This study has provided valuable insight into what different aspects of data quality exist for a sensor network and how they can be assessed.
Accuracy
In addition, a survey among owners of different sensor networks has been conducted. This survey aims at understanding how network owners currently assess the quality of their data. From this survey it became clear that data quality is an important aspect for the different applications, but that different aspects of data quality are important for the individual use case. For example, in some cases it is very important that the data is highly accurate, whereas in case of a large-scale sensor network with low-cost sensors, the accuracy is less relevant.
The survey also revealed that data redundancy is often not considered in the different applications. Whether this is because redundancy is deemed irrelevant, or whether it is because this is a relatively unknown aspect of data quality in sensor networks, remains unclear at this stage.