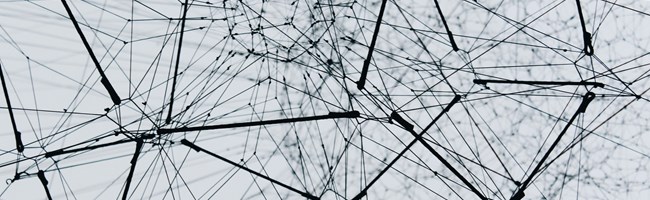
Measurements of sensor networks outside laboratory conditions are complex and call for uncertainty evaluations
The measurement challenges in many emergent areas related to climate and environment, energy, and others, involve measurements outside laboratory conditions where – contrary to the lab conditions - the responses have not been validated by well-established methods to ensure proper traceability. In such cases, the measurement models need to be modified to accommodate the behaviour of the sensors outside laboratory conditions so that concepts of traceability, uncertainty evaluation and calibration can also apply in those more complex conditions.
Those more complex conditions arise because we no longer fully understand all aspects of the response of the more complex system, which include the number of sensors required to characterize the system to be measured, and still obtain accurate information on that system. What would be the response if one or more sensors become faulty, just to mention typical issues in sensor networks. Among other things, there will be issues related to temporal and spatial correlation which should be considered, to allow for the estimation of the measurand and its associated uncertainty.
Uncertainty quantification
In sensor networks, concepts of uncertainty quantification arise. In the sensor network-setting, the classical approaches described in JCGM 100:2008 "Guide to the expression of uncertainty in measurement” (GUM) will be of limited value, as this guide is mainly aimed at univariate explicit formulation typical of most calibration situations. The GUM uncertainty framework was intended to propagate information about input quantities to information about the measurand (one or more). For sensor networks, we need to use regression methods to learn the correlation behaviour from measured data collected at different locations and to use this knowledge for prediction or interpolation within an area of interest.
Learning the correlation behaviour
Gaussian Process (GP) regression is a flexible way of modelling data that is not limited by a functional form. GPs are typically used to model a variable that has spatial and/or temporal dependence. They do so by regarding the values of the variable at different spatial locations as being correlated and describing the strength of the correlation in a flexible way as a function of spatial separation. The idea of GP regression is to learn the correlation behaviour from measured data collected at known, fixed locations. This knowledge can be used for prediction/interpolation within an area of interest. An advantage of GP regression is that the uncertainties associated with predicted values are automatically provided, as they are a Bayesian approach.